Bayesian analysis of road accidents: a general framework for the multinomial case
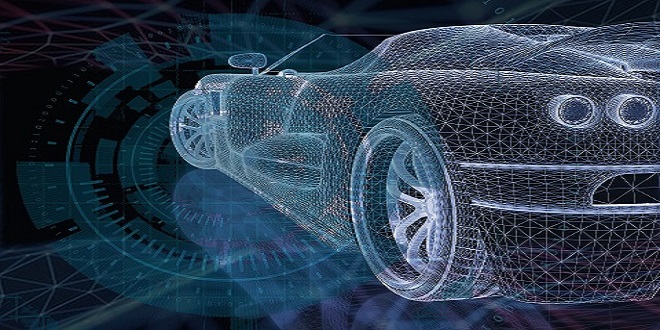
Introduction
An important aspect in road safety research concerns the development of analytical tools to identify road sites with high risk. Within a context of optimization subject to financial constraints, decisions have to be taken as to which sites should be considered for treatment or safety improvement. The most economically reasonable selection criterion is to select those sites which had the highest accident rate in the preceding year.
This is a bad procedure because of the well-known regression to the mean problem. Even if no remedial treatment is made, the number of accidents recorded at the same site in the following year will naturally decrease toward its temporal mean. In other word, very high accident rates should be viewed as outliers.
Standard analyses of accident proportions
n this section we describe a standard EB approach to study accident proportions. We call standard an approach that is correct and simple to implement, but which makes restrictive distributional assumptions about the process generating the data. The more general versions considered in Section 4.3, are more computer-intensive but allow for a lot more flexibility and realism.
In particular, two types of heterogeneity and spatial correlation are assumed to be potentially present. For the convenience of the reader, we first review the original approach of He decker and Wu (1991) which is formulated for the binomial case. Then, we proceed with the extension that we propose for the multinomial case. This first extension will still be viewed as standard because of the assumptions made.
Bayesian Analysis
He Bayesian analysis is performed using the posterior distribution of a evaluated at the maximum likelihood (ML) estimates a and ~ . The posterior distribution represents the state of knowledge concerning a after the observations (XI’ … , Xl) have been combined with the prior information. The Bayesian estimator of the accident proportion at site i is given by the posterior mean:
The Multinomial Case
We now proceed with an extension to the multinomial case of the approach just described. In the binomial approach, the data is assumed to be binomial while the mean parameter 8 is assumed to be beta distributed. The extension involves the use of the multinomial distribution for the accident data and of the Dirichlet distribution for the parameter e which in this case, is a vector.
For convenience, we put in the Appendix the main distributional properties associated with those two distributions.
Our empirical Bayes implementation suggests to retain the valued of a vector. given the I observations of the K-dimensional vector X., i = 1, “” I I, As starting values for an in this estimation process or even as an alternative estimation, one could use the solution of a method of moments (MM) applied on the following relationships (or a subset of it)
Last word
which corresponds to the average Bli value obtained when using all possible values of 9/, not only the median vector (9~ … ,9;). Of course, except for cases with K ~ 2, this integral of dimension 2K would have to be simulated. The numerical complexities associated with the computation give Bajaj an advantage over B2i’ This statement will be reinforced in the general version with heterogeneity and spatial correlation, that we now describe.