Heterogeneous models and prediction on longitudinal data
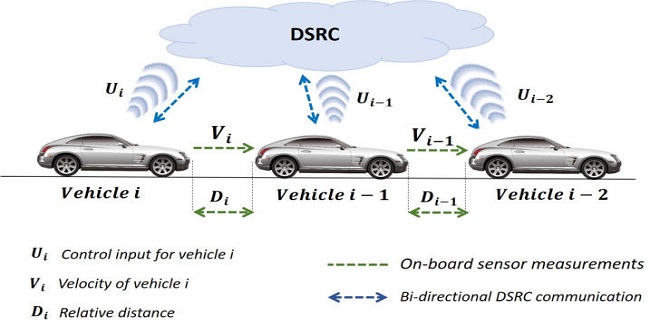
Let us suppose an individual observed on T periods: Yr. == (Pym …, YT) is the sequence of severity variables, and .x;. == (XP···,xT) that of the covariates. The sequences .x;. and Yr take the place of Xj and Yj in the preceding sections. The date of forecast T must be given here, and the individual index can be suppressed since the policyholder can be considered separately. Besides, belonging to the working sample is not mandatory for this policyholder.
We want to predict risk for the period T + I, by means of a heterogeneous model. For the period t, this risk R, is the expectation of a function of Y, (Y, is the outcome of Y,). We now include a heterogeneity component u( The distribution of Y, conditional on u, depends on 9 1, x, and u( This applies to R” and we can write R, = hs,(x) g(u,), for the three types of risk dealt with later (frequency of claims, expected cost per claim, pure premium), g being a real-valued function.
Examples of prediction through heterogeneous models
We give here examples of explicit predictions that are derived from models presented before. The prediction formula given in (4) can be used in any case. provided we have consistent estimators for the heterogeneous model.
The generalized negative binomial model for a number of claims
We derive here bonus-malus coefficients for expected cost per claim. Performing this only through the heterogeneous model on cost distributions supposes the independence between the random effects in the equations related to number and cost of claims. The bonus-malus coefficients will depend on the relative severity of the claims. For instance. a cost bonus will appear after the ftrst claim if its cost is inferior to the estimation made by the rating model.
The log-normal model for the cost of claims
if we suppose that 1fE ::: 1;’E. They have indeed the same limit (see Hausman, 1978, 1984 for a test of random effects vs. fixed effects in linear and Poisson models). Notice that flrE can be seen as an individual “loss to premium” ratio if losses are measured by the number of claims.
Comparison with actual bonus-malus systems
Let us consider for instance the official rules of computation for bonus-malus coefficients in France. A new driver begins with a bonus-malus coefficient equal to one, and this coefficient is equal to 0.95 after one year if no claim with liability is reported. The coefficient is equal to (1.25)n if n claims with liability are reported during the first year, and is bounded by 3.5. Suppose that the estimated frequency of the claims reported by the new driver is equal to 0.1. If we express the bonus-malus coefficients as weighted averages of the preceding type, we obtain
statistical methods that can be used for the estimation of heterogeneous models that are recalled in this section. The following section presents a method developed by the author for these models. Maximum likelihood estimation (m.l.e.) of parameterized models is the basic way to describe a data generating process. We recall its convergence properties in a misspecification context.
Last word
As an example of a pseudo-true value, consider (P m)meM’ a family of equivalent distributions, parameterized by the expectation (m = Ep (ld), where Id stands for the identity on the support of Pm). For instance, M = 1R+ for a P’;,Poisson distribution, and M = [0,1] for a distribution on {O, I}. Suppose that the densities with respect to an equivalent measure, 11, have a linear exponential structure, i.e